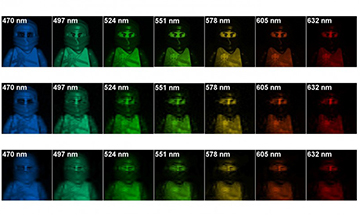
Images from 470 nm to 632 nm reconstructed by the new algorithm and another state-of-art algorithm. Top: ground truth; middle: output of new algorithm; bottom: output of the other algorithm. Credit: Dror Baron / NC State Univ [Enlarge image]
Hyperspectral imaging benefits many fields of research, from medical imaging to environmental monitoring, but acquiring and transmitting the large amounts of raw data generated by the imaging technique can be cumbersome in practice, and data-compression algorithms can require lengthy reconstruction procedures at the other end of the pipeline.
Researchers at two U.S. universities have devised a new, faster image-reconstruction algorithm for hyperspectral-image data sets (IEEE J. Sel. Topics in Signal Processing, doi: 10.1109/JSTSP.2015.2500190). The scientists took an iterative reconstruction algorithm for two-dimensional images, extended it to three dimensions and added a damping method to allow the results to converge.
Specifically, the team from North Carolina State University (NCSU) and the University of Delaware examined data from a coded aperture snapshot spectral imager (CASSI), which uses a 2-D array to measure 3-D image cubes and thus acquires far fewer data points than conventional spectral imagers. Because CASSI data are highly compressed, 3-D image reconstruction becomes a challenge.
The researchers, led by NCSU professor Dror Baron, applied a technique called approximate message passing (AMP) to the CASSI data. AMP removes noise from data at each iteration of the algorithm; the team chose an adaptive Wiener filter for the noise reduction. To generate sample data, the scientists imaged a plastic toy under wide-band lighting in the laboratory. The resulting data cube contained 256 x 256 pixels of spatial resolution and 24 9-nm-wide spectral bands.
Testing their method against existing image-reconstruction techniques that require lots of parameter tweaking, the NCSU-Delaware group found that their algorithmic combination outperformed the others—it produced sharper results after 100 s of runtime than other techniques produced in 450 s. Unlike the different techniques, which need to be run multiple times with varying parameters, the group's combo worked on the first try. The results held true for two “natural scene” data sets that had more spectral bands and higher spatial resolution than the images of the plastic toy.
In the future, team members want to improve the noise reduction within their algorithm and possibly remove the complementarity constraint on coded apertures.